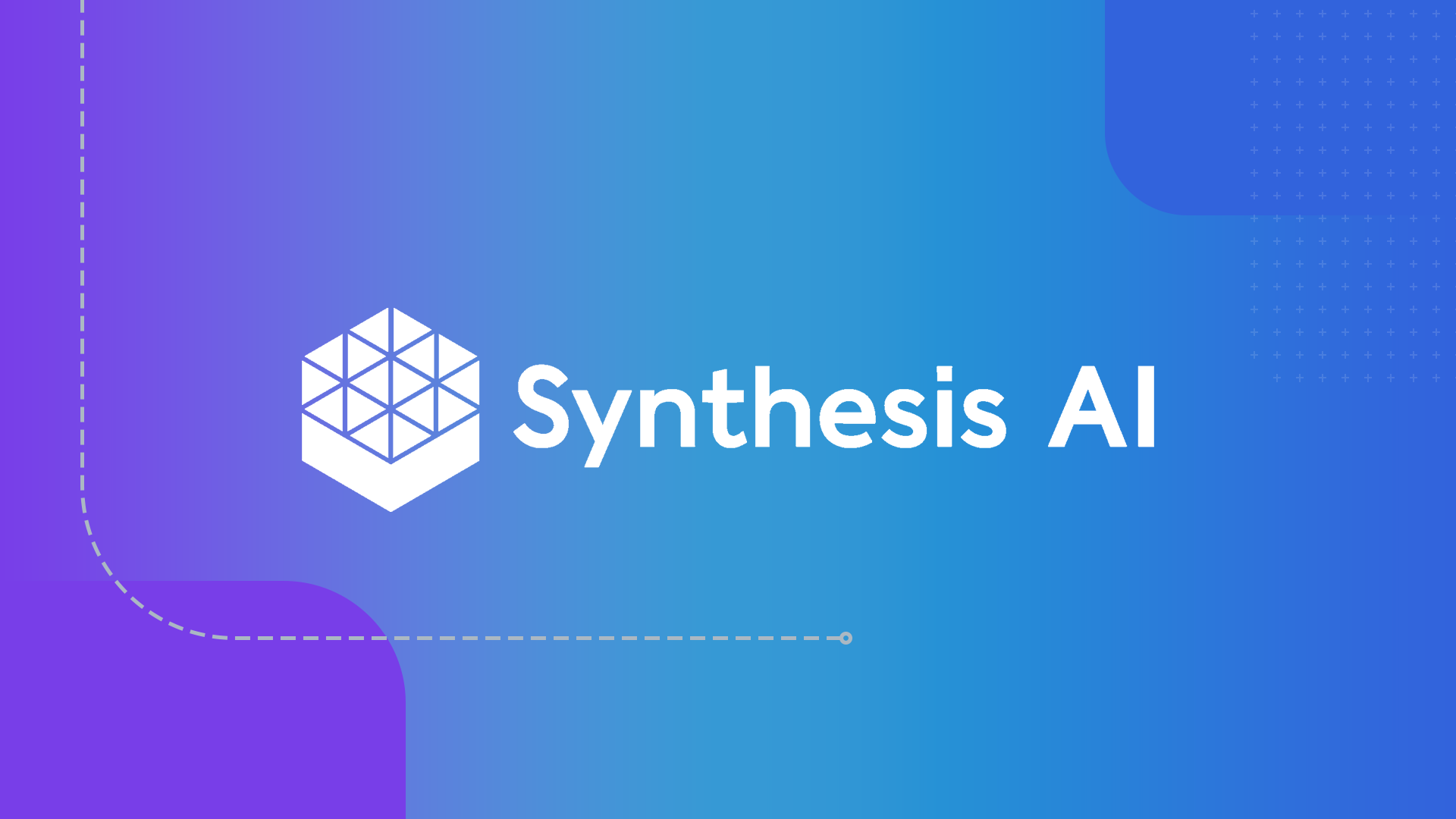
For all of the recent hype around generative AI models like Chat GPT and DALL-E, AI progress in many real-world applications can still be painfully slow. This is especially true in enterprise environments where AI models frequently fail to graduate from proofs of concept to commercial, production-scale use cases.
Although a variety of factors contribute to the difficulties companies have in implementing production-scale AI models—lack of technical capabilities, cost, and human labeling accuracy and bias issues to name a few—the ability to generate and use high-quality data to train models is often cited as the key bottleneck that blocks AI progress. In fact, a recent Gartner study found that nearly half of global enterprises–46%–failed to move AI models from proof of concept to production due to challenges associated with the production of high-quality, real-world data.
That’s why we were so excited when we met Yashar Behzadi, the CEO and Founder of Synthesis AI. Synthesis AI is a San Francisco-based synthetic data startup that literally wrote the book, Synthetic Data for Deep Learning, on how manufactured data can be used to improve AI model performance in both academic and industry settings.
Synthesis AI’s team combines experts from generative AI and cinematic visual effects. Together they have figured out how to produce large volumes of high-quality images and video that can be used to train AI systems that rely on a precise understanding of how humans interact with physical environments.
Because it’s synthetic, Synthesis AI data is unlimited in nature, available on-demand, photo-realistic, pixel perfect, diverse, accurately pre-labeled, and bias free. What’s more, Synthesis AI provides customers comprehensive and fine-grained control over how training data is generated. This means Synthesis customers can create an infinite number of variations to meet the specific needs for each use case.
From a business perspective, Synthesis AI enables customers to produce high-quality, perfectly pre-labeled data 100x faster and at 20x lower cost than competitive solutions. Not to mention the ML models built with Synthesis AI-generated data perform better than models developed with real-world data, while avoiding the common legal, ethical, and regulatory consumer privacy issues created by human data and labeling bias.
What’s most unique about Synthesis AI, however, is the way the company’s 3D models combine techniques from computer vision and cinematic visual effects to account for the physics of light, human and environmental physical attributes, and hardware and device characteristics for relevant capture devices and sensors, regardless of sensor and capture device type. Synthesis AI, for example, can handle image and video cameras, X-Ray, and LIDAR. They also factor in critical device characteristics like lens model, focal length, and sensor type to ensure their synthetic data output is photorealistic.
Perhaps, most importantly, Synthesis AI addresses environmental inconsistencies in a highly consistent manner. In simple terms, this means that Synthesis AI is able to produce large volumes of high-quality, on-demand synthetic data to help companies predict human and environmental interactions accurately and precisely, reducing risk and preventing potentially dangerous situations for humans.
That’s why Synthesis AI has been able to attract a large portfolio of customers, representing some of the world’s most recognized and respected global brands. Companies like Apple, Google, Ford, John Deere, and Amazon all rely on Synthesis AI for high volumes of synthetic image and video data that they use to train AI models. Example customer use cases include security and smart cities, autonomous robotics and vehicles, ID verification, AR/VR, and driver monitoring.
Although we’re impressed by what the Synthesis AI team has already accomplished, we’re even more excited about the company’s enormous potential. As Synthesis expands its capabilities beyond human-centered synthetic data generation to increasingly complex scenarios—think multiple humans in complex environments and large-scale simulations with behaviorally accurate agents and crowds–we believe Yashar and team will enable rapid-growth in large new markets, including gaming, visual effects, and simulation. Not to mention that Synthesis will soon support the use of simple text to define 3D asset creation, making the use of synthetic data easier and more accessible than ever before.
Just like Snowflake achieved tremendous success by making data available across environments and making sophisticated data analytics easy and accessible, we believe Synthesis AI will play a similar role in the AI industry. Synthesis makes it easy for enterprises to produce the data they need to train AI models, accelerating market growth across the industry.
We’re very excited to work with Yashar and the Synthesis AI team as they help enterprises deploy better AI models faster with synthetic data.